Risk Prediction of Early Chronic Kidney Disease: Net Benefit in Individuals with Type 2 Diabetes.
Dunkler D (1,2,3), Gao P (1), Fu Lee S (1), Heinze G (3), Clase CM (4), Tobe S (5), Teo KK (1,4), Gerstein H (1), Mann JFE (2,6), Oberbauer R (3,7), on behalf of the ONTARGET & ORIGIN Investigators
1- Population Health Research Institute, Hamilton Health Sciences/McMaster University, Hamilton, Ontario, Canada
2- Universitaetsklinikum Erlangen, Department of Nephrology, Germany
3- Section for Clinical Biometrics, Center for Medical Statistics, Informatics and Intelligent Systems, Medical University of Vienna, Austria
4- McMaster University, Hamilton, Ontario, Canada
5- Sunnybrook Health Sciences Center, Toronto, Ontario, Canada
6- Schwabing General Hospital, and KfH Kidney Center, Munich, Germany
7- KH Elisabethinen Linz, Austria, and Department of Internal Medicine III, Medical University of Vienna, Austria
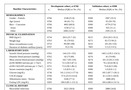
Baseline characteristics of participants with diabetes in the development and validation cohorts. Median (interquartile-range, IQR) or frequencies (%) are given.
View PDF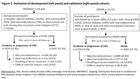
Derivation of development (left panel) and validation (right panel) cohorts.
View PDF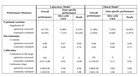
Performance of prediction models in the development and validation cohorts.
Optimism-corrected performance measures were computed by internal validation with 500 bootstrap resamples. Results for the outcome ‘alive without incidence and progression of CKD’ (CKD) and ROC curves can be found in Supplemental Table 3 and Supplemental Figure 3, respectively.
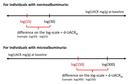
Explanation of the predictor d-UACRtp. Micro- and macroalbuminuria were defined as urinary-albumin creatinine ratio (UACR) above 30 mg/g (3.4 mg/mmol) and 300 mg/g (33.9 mg/mmol), respectively. To predict incidence or progression of chronic kidney disease (CKD) after 5.5 years the current (at baseline) UACR measurement is clearly an important predictor. Therefore, for each participant, we calculated d-UACRtp as the difference between each participant’s UACR at baseline and the cutoff that was relevant for this participant. For individuals with normoalbuminuria at baseline [UACR<30 mg/g] the relevant cutoff for incidence of albuminuria is 30 mg/g and for individuals with microalbuminuria at baseline [30
[/data-info-col-1]

Predicted probabilities for incidence or progression of chronic kidney disease after 5.5 years and death within 5.5 years computed by the laboratory model. For a hypothetical male, 65-year old individual with normoalbuminuria (top panels) or with microalbuminuria (bottom panels) predicted probabilities were computed by inserting eGFR CKD-EPI values and selected UACR values into the laboratory model. The plots reveal that the predicted probability for death within 5.5 years is higher in an individual with microalbuminuria at baseline than in an individual with normoalbuminuria. As a consequence, an individual with microalbuminuria at baseline has a lower predicted probability for CKD after 5.5 years than an individual with normoalbuminuria.
View PDF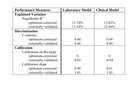
Performance of Prediction Models in the Development and Validation Cohorts for the Outcome ‘Alive without incidence or progression of chronic kidney disease.
View PDF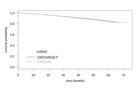
Supplemental Figure 1. Kaplan‐Meier Plot Comparing Follow‐Up of ONTARGET and ORIGIN
View PDF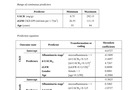
Prediction equations:
(a) Laboratory Prediction Equation, (b) Clinical Prediction Equation
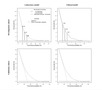
Decision curve analysis for the laboratory (left panel) and the clinical model (right panel) for the outcome state ‘alive with incidence and progression of CKD’ based on the development cohort (first row) and the validation cohort (second row).
View PDF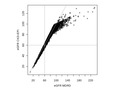
Scatterplot comparing eGFR CKD‐EPI and eGFR MDRD
View PDF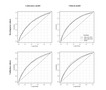
Receiver‐Operating Curves (ROC) for the Laboratory and the Clinical Model based on the Development and Validation Cohort.
View PDF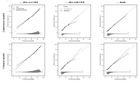
Calibration plots
View PDF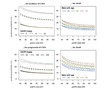
Predicted Probabilities for incidence or progression of chronic kidney disease after 5.5 years and death within 5.5 years computed by the laboratory model.
(a) Hypothetical male individual aged 60, 65 or 70.
(b) Hypothetical female individual aged 60, 65 or 70.